The amount of medically relevant sensitive data is growing rapidly and based on machine learning methods (so-called AI methods), this data can be processed more and more usefully. However, these learning methods reveal information about the sensitive medical data that was processed with them. So how can medical data be processed usefully and at the same time protect sensitive patient information? Research has made promising progress in recent years on this so-called privacy problem; however, a lot of research is still needed for most medical applications. This is exactly where AnoMed comes in as one of five nationwide anonymization competence clusters funded by the BMBF and the EU.
As part of the AnoMed project, the researchers are presenting an interactive board game. In it, they playfully demonstrate the threats to privacy that arise from training AI models. The game is based on cards with personal information about people, such as occupation, interests, and household situation. From these cards, a player selects 5 cards and "trains a model": The attributes directly result in the "model parameters" as positions of game pieces. The other players, as attackers, then try to guess from the positions of the game pieces what the private attributes of the cards were exactly.
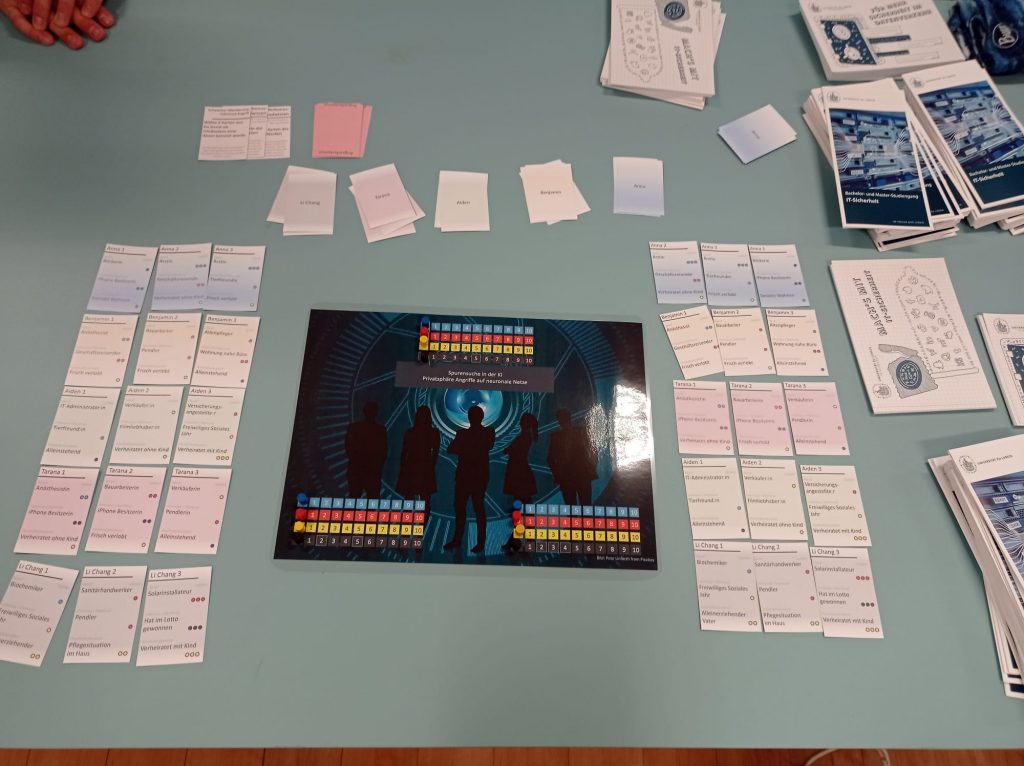
The playfully simple representation shows one of the known dangers of complex AI models: even if the parameters of the models may be incomprehensible to a human at first glance, they can still contain sensitive information about the training data. Sufficiently skilled or resourceful attackers can read such information from the model. Although the amount of training data is much larger in practice (millions of data points, instead of just 5 out of 15 playing cards), the models also have a much larger number of parameters (millions of parameters instead of 4 colors). With the game, the researchers hope to open a dialogue that both arouses a basic interest in the topic and provides a platform for more detailed discussions.